Addressing Bias in Facial Recognition: Balancing Accuracy and Ethics
Written on
Understanding Facial Recognition Technology
In recent years, the use of facial recognition technology has surged dramatically. You might be familiar with systems that identify individuals, such as Facebook's photo-tagging feature or Apple's FaceID. There are also detection systems that simply check for the presence of a face, while analysis systems aim to discern attributes like gender and ethnicity. These technologies are being employed across various sectors, from recruitment to security and surveillance.
Many assume that these systems are both highly effective and unbiased. The prevailing belief is that, unlike human operators who may experience fatigue or bias, a well-trained AI should consistently and accurately identify any face. However, extensive research reveals that these systems often perform poorly for certain demographic groups.
For instance, last year, the Gender Shades project, spearheaded by MIT Media Lab's Joy Buolamwini, uncovered that gender classification systems from IBM, Microsoft, and Face++ exhibited error rates up to 34.4 percentage points higher when identifying darker-skinned women compared to lighter-skinned men. Similarly, the ACLU of Northern California found that Amazon's platform was more prone to misidentifying non-white members of Congress.
The root of the issue lies in the biased data sets used to train these systems. They often contain significantly fewer images of women and individuals with darker skin compared to men and those with lighter skin. While many systems claim to undergo fairness testing, these assessments often fail to evaluate a comprehensive range of faces, as demonstrated by Buolamwini's findings. Such disparities perpetuate existing inequalities and can have serious consequences, especially in high-stakes environments.
Recent Research on Bias Mitigation
Three new studies released in the past week are shedding light on this pressing issue. Below are summaries of each paper.
Paper 1: Gender Shades Update
Last Thursday, Buolamwini published an updated analysis of Gender Shades, retesting previously examined systems and incorporating Amazon's Rekognition and a new solution from a smaller AI firm, Kairos. The findings are encouraging: IBM, Face++, and Microsoft have enhanced their accuracy for identifying darker-skinned women, with Microsoft reducing its error rate to under 2%. However, Amazon's and Kairos's systems still exhibit significant accuracy gaps of 31 and 23 percentage points, respectively, when comparing lighter males to darker females. Buolamwini emphasized the necessity for external audits to ensure accountability in these technologies.
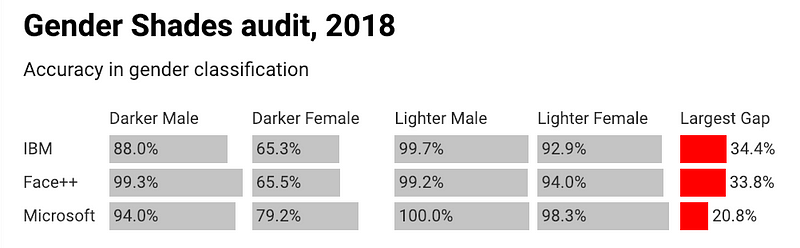
Paper 2: Bias Mitigation Algorithm
On Sunday, a team from MIT's Computer Science and Artificial Intelligence Laboratory (CSAIL) introduced a novel algorithm capable of reducing biases in face detection systems, even when trained on significantly biased data. The algorithm identifies underrepresented examples in the dataset and allocates additional training time to these instances. When tested against Buolamwini's Gender Shades dataset, it managed to reduce the largest accuracy gap between lighter and darker-skinned males, although it did not eliminate the gap entirely.
Paper 3: Measuring Facial Diversity
This morning, IBM Research released a paper outlining various features for assessing diversity beyond just skin color and gender, including head height, face width, intra-eye distance, and age. "Without measures of facial diversity," asserts coauthor John Smith, "we cannot ensure fairness as we train these recognition systems." Alongside this research, the team has made available a new dataset of one million annotated images to facilitate the exploration of these measures.
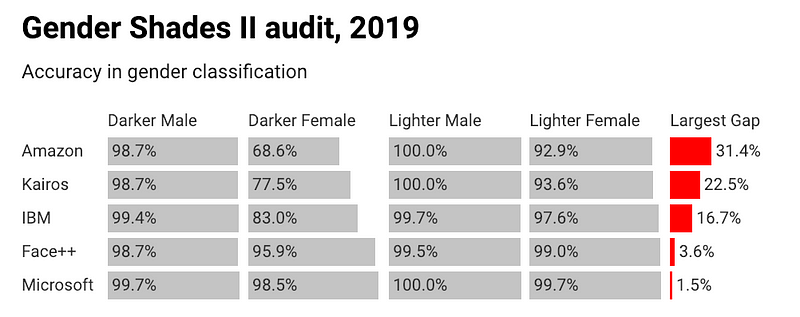
Balancing Accuracy and Ethical Use
While these studies represent significant progress in mitigating bias in facial recognition, they also highlight a critical issue: even the most equitable and accurate systems can still infringe upon civil liberties. Investigations by the Daily Beast revealed that Amazon was actively promoting its facial recognition technology to the U.S. Immigration and Customs Enforcement (ICE) for monitoring migrant communities. Furthermore, the Intercept uncovered IBM's partnership with the New York Police Department, which involved developing ethnicity identification technology for deployment in public surveillance without community awareness.
Currently, the UK Metropolitan Police utilize facial recognition to scan public crowds for individuals on watch lists, and in China, this technology is employed for mass surveillance, including tracking dissenters. In light of these developments, an increasing number of civil rights advocates and technologists are calling for stringent regulations on facial recognition systems. Google has even paused the sale of such technologies until it devises clear strategies to prevent their misuse.
"Without algorithmic justice, achieving algorithmic accuracy and technical fairness may result in AI tools that are weaponized," warns Buolamwini.
Karen Hao serves as the artificial intelligence reporter for MIT Technology Review, focusing on the ethical implications and societal impacts of technology, as well as its potential for positive social change. She also authors the AI newsletter, the Algorithm.
To receive this story directly in your inbox, subscribe to the Algorithm here for free.
Chapter 2: Video Insights
The first video, "The Creepy Facial Recognition Technology Changing Law Enforcement," explores the implications of facial recognition technologies and their impact on law enforcement practices.
The second video, "Revealing Hidden Biases in Face Representation via Deceptively Simple Tasks," delves into the biases present in face recognition algorithms and how they can be addressed.